网络结构
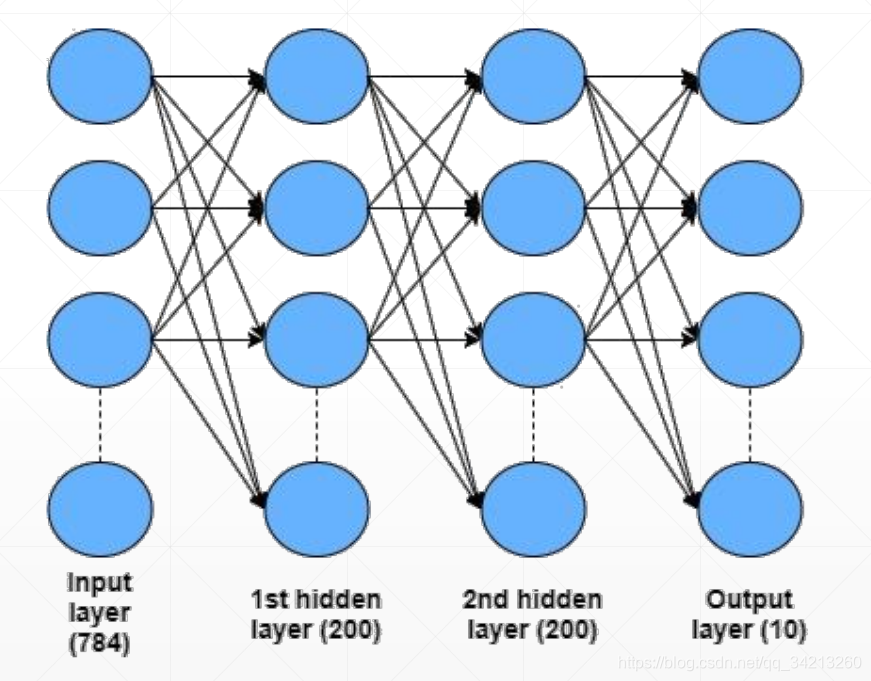
代码
import torch
import torch.nn as nn
import torch.nn.functional as F
import torch.optim as optim
from torchvision import datasets, transforms
batch_size=200
learning_rate=0.01
epochs=10
# 下载数据
train_loader = torch.utils.data.DataLoader(
datasets.MNIST('../data', train=True, download=True,
transform=transforms.Compose([
transforms.ToTensor(),
transforms.Normalize((0.1307,), (0.3081,))
])),
batch_size=batch_size, shuffle=True)
test_loader = torch.utils.data.DataLoader(
datasets.MNIST('../data', train=False, transform=transforms.Compose([
transforms.ToTensor(),
transforms.Normalize((0.1307,), (0.3081,))
])),
batch_size=batch_size, shuffle=True)
w1, b1 = torch.randn(200, 784, requires_grad=True),
torch.zeros(200, requires_grad=True)
w2, b2 = torch.randn(200, 200, requires_grad=True),
torch.zeros(200, requires_grad=True)
w3, b3 = torch.randn(10, 200, requires_grad=True),
torch.zeros(10, requires_grad=True)
torch.nn.init.kaiming_normal_(w1)
torch.nn.init.kaiming_normal_(w2)
torch.nn.init.kaiming_normal_(w3)
#自己定义结构实现
def forward(x):
x = x@w1.t() + b1
x = F.relu(x)
x = x@w2.t() + b2
x = F.relu(x)
x = x@w3.t() + b3
x = F.relu(x)
return x
# 使用Pytorch的API实现
class MLP(nn.Module):
def __init__(self):
super(MLP, self).__init__()
self.model = nn.Sequential(
nn.Linear(784, 200),
nn.ReLU(inplace=True),
nn.Linear(200, 200),
nn.ReLU(inplace=True),
nn.Linear(200, 10),
nn.ReLU(inplace=True),
)
def forward(self, x):
x = self.model(x)
return x
# GPU加速
device = torch.device('cuda:0')
net = MLP().to(device)
# 优化方法SGD 待优化变量 [w1, b1, w2, b2, w3, b3]
# optimizer = optim.SGD([w1, b1, w2, b2, w3, b3], lr=learning_rate)#自己定义结构实现
optimizer = optim.SGD(net.parameters(), lr=learning_rate)# 使用Pytorch的API实现
# loss_function 交叉熵
criteon = nn.CrossEntropyLoss().to(device)
for epoch in range(epochs):
for batch_idx, (data, target) in enumerate(train_loader):
# 重构为x*28*28的尺寸 28*28=784
data = data.view(-1, 28*28)
# GPU加速
data, target = data.to(device), target.cuda()
# 网络结构
# logits = forward(data)#自己定义结构实现
logits = net(data)# 使用Pytorch的API实现
# 计算损失函数
loss = criteon(logits, target)
# 初始化梯度为0
optimizer.zero_grad()
# 计算反向传播梯度
loss.backward()
# print(w1.grad.norm(), w2.grad.norm())
# 进行一次优化更新
optimizer.step()
if batch_idx % 100 == 0:
print('Train Epoch: {} [{}/{} ({:.0f}%)] Loss: {:.6f}'.format(
epoch, batch_idx * len(data), len(train_loader.dataset),
100. * batch_idx / len(train_loader), loss.item()))
test_loss = 0
correct = 0
for data, target in test_loader:
data = data.view(-1, 28 * 28)
data, target = data.to(device), target.cuda()
# logits = forward(data)
logits = net(data)
test_loss += criteon(logits, target).item()
pred = logits.data.max(1)[1]
correct += pred.eq(target.data).sum()
test_loss /= len(test_loader.dataset)
print('
Test set: Average loss: {:.4f}, Accuracy: {}/{} ({:.0f}%)
'.format(
test_loss, correct, len(test_loader.dataset),
100. * correct / len(test_loader.dataset)))