import pandas as pd
import numpy as np
from sklearn.model_selection import train_test_split
from sklearn.tree import DecisionTreeClassifier
import seaborn as sns
from sklearn.metrics import confusion_matrix
from matplotlib import pyplot as plt
from sklearn import tree
from sklearn.metrics import accuracy_score
from sklearn.linear_model import LogisticRegression
from sklearn.naive_bayes import GaussianNB
from sklearn.ensemble import RandomForestClassifier, VotingClassifier
from sklearn import svm
# 读取数据
data_load = "bankloan.xls"
data = pd.read_excel(data_load)
data.describe()
|
年龄 |
教育 |
工龄 |
地址 |
收入 |
负债率 |
信用卡负债 |
其他负债 |
违约 |
count |
700.000000 |
700.000000 |
700.000000 |
700.000000 |
700.000000 |
700.000000 |
700.000000 |
700.000000 |
700.000000 |
mean |
34.860000 |
1.722857 |
8.388571 |
8.278571 |
45.601429 |
10.260571 |
1.553553 |
3.058209 |
0.261429 |
std |
7.997342 |
0.928206 |
6.658039 |
6.824877 |
36.814226 |
6.827234 |
2.117197 |
3.287555 |
0.439727 |
min |
20.000000 |
1.000000 |
0.000000 |
0.000000 |
14.000000 |
0.400000 |
0.011696 |
0.045584 |
0.000000 |
25% |
29.000000 |
1.000000 |
3.000000 |
3.000000 |
24.000000 |
5.000000 |
0.369059 |
1.044178 |
0.000000 |
50% |
34.000000 |
1.000000 |
7.000000 |
7.000000 |
34.000000 |
8.600000 |
0.854869 |
1.987568 |
0.000000 |
75% |
40.000000 |
2.000000 |
12.000000 |
12.000000 |
55.000000 |
14.125000 |
1.901955 |
3.923065 |
1.000000 |
max |
56.000000 |
5.000000 |
31.000000 |
34.000000 |
446.000000 |
41.300000 |
20.561310 |
27.033600 |
1.000000 |
data.columns
Index(['年龄', '教育', '工龄', '地址', '收入', '负债率', '信用卡负债', '其他负债', '违约'], dtype='object')
data.index
RangeIndex(start=0, stop=700, step=1)
## 转为np 数据切割
X = np.array(data.iloc[:,0:-1])
y = np.array(data.iloc[:,-1])
X_train, X_test, y_train, y_test = train_test_split(X, y, random_state=1, train_size=0.8, test_size=0.2, shuffle=True)
决策树
Dtree = DecisionTreeClassifier(max_leaf_nodes=3,random_state=13)
Dtree.fit(X_train, y_train)
y_pred = Dtree.predict(X_test)
accuracy_score(y_test, y_pred)
0.7285714285714285
tree.plot_tree(Dtree)
plt.show()
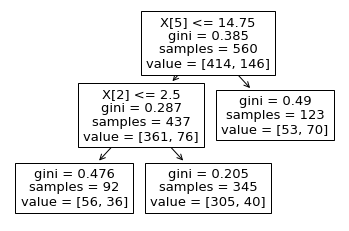
## seaborn
cm = confusion_matrix(y_test, y_pred)
heatmap = sns.heatmap(cm, annot=True, fmt='d')
heatmap.yaxis.set_ticklabels(heatmap.yaxis.get_ticklabels(), rotation=0, ha='right')
heatmap.xaxis.set_ticklabels(heatmap.xaxis.get_ticklabels(), rotation=45, ha='right')
plt.ylabel("true label")
plt.xlabel("predict label")
plt.show()
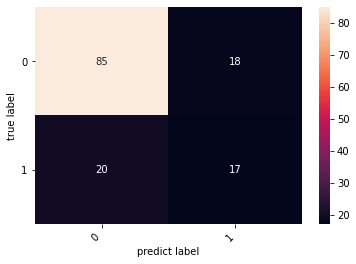
SVM
svm = svm.SVC()
svm.fit(X_test,y_test)
y_pred = svm.predict(X_test)
accuracy_score(y_test, y_pred)
0.7857142857142857
cm = confusion_matrix(y_test, y_pred)
heatmap = sns.heatmap(cm, annot=True, fmt='d')
heatmap.yaxis.set_ticklabels(heatmap.yaxis.get_ticklabels(), rotation=0, ha='right')
heatmap.xaxis.set_ticklabels(heatmap.xaxis.get_ticklabels(), rotation=45, ha='right')
plt.ylabel("true label")
plt.xlabel("predict label")
plt.show()
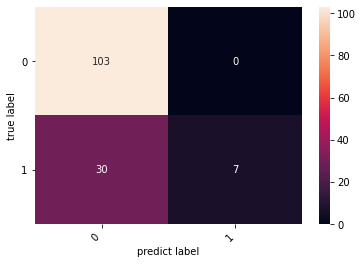
ensemble
# ensemble
from sklearn import svm
clf1 = LogisticRegression(multi_class='multinomial', random_state=1)
clf2 = RandomForestClassifier(n_estimators=50, random_state=1)
clf3 = GaussianNB()
clf4 = svm.SVC()
clf5 = DecisionTreeClassifier(max_leaf_nodes=3,random_state=13)
eclf = VotingClassifier(estimators=[
('lr', clf1), ('rf', clf2), ('gnb', clf3), ('svm', clf4), ('dtree', clf5)], voting='hard')
eclf = eclf.fit(X, y)
y_pred = eclf.predict(X_test)
accuracy_score(y_test, y_pred)
0.8357142857142857
cm = confusion_matrix(y_test, y_pred)
heatmap = sns.heatmap(cm, annot=True, fmt='d')
heatmap.yaxis.set_ticklabels(heatmap.yaxis.get_ticklabels(), rotation=0, ha='right')
heatmap.xaxis.set_ticklabels(heatmap.xaxis.get_ticklabels(), rotation=45, ha='right')
plt.ylabel("true label")
plt.xlabel("predict label")
plt.show()
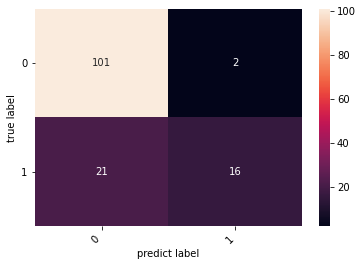
TORCH_BP_NETWORK
import torch
import torch.nn.functional as Fun
train_x = torch.FloatTensor(X_train)
train_y = torch.LongTensor(y_train)
test_x = torch.FloatTensor(X_test)
test_y = torch.LongTensor(y_test)
# BP
class Net(torch.nn.Module):
def __init__(self, n_feature, n_hidden, n_output):
super(Net, self).__init__()
self.hidden = torch.nn.Linear(n_feature, n_hidden) # 定义隐藏层网络
self.out = torch.nn.Linear(n_hidden, n_output) # 定义输出层网络
def forward(self, x):
x = Fun.relu(self.hidden(x)) # 隐藏层的激活函数,采用relu,也可以采用sigmod,tanh
x = self.out(x) # 输出层不用激活函数
return x
net = Net(n_feature=8,n_hidden=20, n_output=2) #n_feature:输入的特征维度,n_hiddenb:神经元个数,n_output:输出的类别个数
optimizer = torch.optim.SGD(net.parameters(), lr=0.02) # 优化器选用随机梯度下降方式
loss_func = torch.nn.CrossEntropyLoss() # 交叉熵损失函数
loss_record = []
for t in range(100):
out = net(train_x) # 输入input,输出out
loss = loss_func(out, train_y) # 输出与label对比
loss_record.append(loss.item())
optimizer.zero_grad() # 梯度清零
loss.backward() # 前馈操作
optimizer.step() # 使用梯度优化器
out = net(test_x) #out是一个计算矩阵,可以用Fun.softmax(out)转化为概率矩阵
prediction = torch.max(out, 1)[1] # 返回index 0返回原值
pred_y = prediction.data.numpy()
target_y = test_y.data.numpy()
accuracy = float((pred_y == target_y).astype(int).sum()) / float(target_y.size)
print(accuracy)
0.8214285714285714
##
ax = sns.lineplot(data = loss_record)
plt.show()
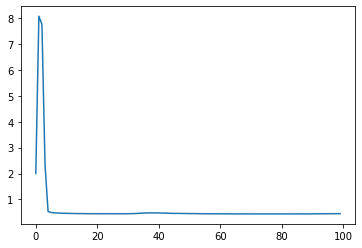
模型读取
torch.save(net, 'net.pt')
netC = torch.load('net.pt')
input = test_x
output = netC(input)
accuracy = float((pred_y == target_y).astype(int).sum()) / float(target_y.size)
print(accuracy)
0.8214285714285714