(for pursue, do accumulation)
个人笔记,纯属佛系分享,如有错误,万望赐教。
动态规划(Dynamic Programming, DP)是基于模型的方法,即在给定一个利用MDP描述的完备的环境模型下可以计算出最优策略的优化算法。
DP的两种性质:1.最优子结构:问题的最优解法可以被分为若干个子问题;2.重叠子问题:子问题之间存在递归关系,解法是可以被重复利用的。在强化学习中,MDP满足两个性质,DP的关键思想就是利用价值函数组织并结构化对好的策略的搜索。
1. 策略评估
策略评估(Policy Evaluation)也被称为“预测问题”,就是计算任意一个随机策略(pi)的状态价值函数(v_pi)的问题。
在MDP中,由公式((2.11))最终得到了状态价值函数的贝尔曼方程:(v_ pi(s)=displaystyle sum_api(a|s) sum_{s^prime.r} p(s^prime,r|s,a) [r+gamma v_pi(s^prime)]),该方程可以通过迭代法求解,方法如下:
1.将状态价值函数序列记为(left{ v_0,v_1,...,v_k
ight})
2.(v_0)作为初始状态价值函数,任意取值(在终止状态时,取值必须为0)
3.通过下面的公式进行迭代$$v_{k+1}=displaystyle sum_api(a|s) sum_{s^prime.r} p(s^prime,r|s,a) [r+gamma v_k(s^prime)] ag{3.1}$$
序列(left{v_k
ight})在(k
ightarrow infty)时将收敛于(v_pi)。该方法需要两个数组:一个用于存储旧的(v_k(s)),另一个用于存储新的(v_{k+1}(s))。也可以每次直接用新状态价值函数替换旧状态价值函数,这就是"in-place"更新。
2. 价值迭代
上述的策略评估方法是一个多次遍历状态集合的迭代过程,因此,可以通过价值迭代(Value Iteration)来缩短策略评估的步骤,公式如下:
通过公式((3.2))可以在一次遍历后立即停止策略评估,只需要对每个状态更新一次,从而提升计算效率。
3. 策略改进
通过策略评估得出策略的状态价值函数,可以根据策略改进定理(policy improvement theorem)选择出贪心策略:
对于任意两个确定策略(pi)和(pi^prime),(forall s in mathcal{S},q_pi(s,pi^prime(s)) geq v_pi(s)),则策略(pi^prime)不劣于(pi)。
在这种情况下,(v_{pi^prime}(s) geq v_pi(s))。证明过程如下
由此,可以推出贪心策略(pi^prime),满足
同时,可以写出它的状态价值函数:
4. 策略迭代
通过下面的链式方法,可以得到一个不断改进的策略和状态价值函数的序列:
(stackrel{E}{longrightarrow})表示策略评估,(stackrel{I}{longrightarrow})表示策略改进,每一次的策略评估都是一个迭代计算的过程,需要基于前一个策略的状态价值函数开始计算。
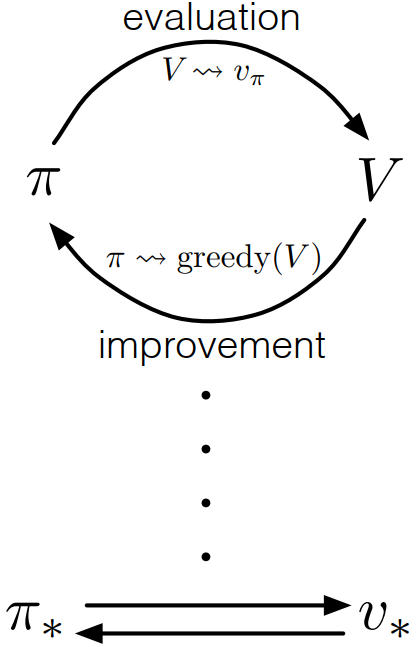

5. 异步动态规划
上述的都是同步动态规划(synchronous dynamic programming),它们的缺点是需要对MDP的整个状态集进行遍历。异步动态规划(Asynchronous Dynamic Programming)使使用任意可用的状态值,以任意规则进行更新,为了确保能够正确收敛,异步动态规划必须不断更新所有状态的值。
(转载请标明出处:https://www.cnblogs.com/HughCai/p/12770811.html)
References
Richard S. Sutton and Andrew G. Barto. Reinforcement Learning: An Introduction (Second Edition). Cambridge, Massachusetts London, England : The MIT Press, 2018.
Csaba Szepesvári, ‘Algorithms for Reinforcement Learning’, Synthesis Lectures on Artificial Intelligence and Machine Learning, vol. 4, no. 1, pp. 1–103, Jan. 2010, doi: 10.2200/S00268ED1V01Y201005AIM009.
UCL Reinforcement Learning Course by David Silver:https://www.bilibili.com/video/BV1b7411y7ax