Convolutional Neural Networks: Step by Step
implement convolutional (CONV) and pooling (POOL) layers in numpy, including both forward propagation and (optionally) backward propagation.
Notation:
-
Superscript ([l]) denotes an object of the (l^{th}) layer.
- Example: (a^{[4]}) is the (4^{th}) layer activation. (W^{[5]}) and (b^{[5]}) are the (5^{th}) layer parameters.
-
Superscript ((i)) denotes an object from the (i^{th}) example.
- Example: (x^{(i)}) is the (i^{th}) training example input.
-
Lowerscript (i) denotes the (i^{th}) entry of a vector.
- Example: (a^{[l]}_i) denotes the (i^{th}) entry of the activations in layer (l), assuming this is a fully connected (FC) layer.
-
(n_H), (n_W) and (n_C) denote respectively the height, width and number of channels of a given layer. If you want to reference a specific layer (l), you can also write (n_H^{[l]}), (n_W^{[l]}), (n_C^{[l]}).
-
(n_{H_{prev}}), (n_{W_{prev}}) and (n_{C_{prev}}) denote respectively the height, width and number of channels of the previous layer. If referencing a specific layer (l), this could also be denoted (n_H^{[l-1]}), (n_W^{[l-1]}), (n_C^{[l-1]}).
1. Packages
import numpy as np
import h5py
import matplotlib.pyplot as plt
%matplotlib inline
plt.rcParams['figure.figsize'] = (5.0, 4.0) # set default size of plots
plt.rcParams['image.interpolation'] = 'nearest'
plt.rcParams['image.cmap'] = 'gray'
%load_ext autoreload
%autoreload 2
np.random.seed(1)
2. Outline of Assignment
-
Convolution functions, including:
-
Zero Padding
-
Convolve window
-
Convolution forward
-
Convolution backward (optional)
-
-
Pooling functions, including:
-
Pooling forward
-
Create mask
-
Distribute value
-
Pooling backward (optional)
-
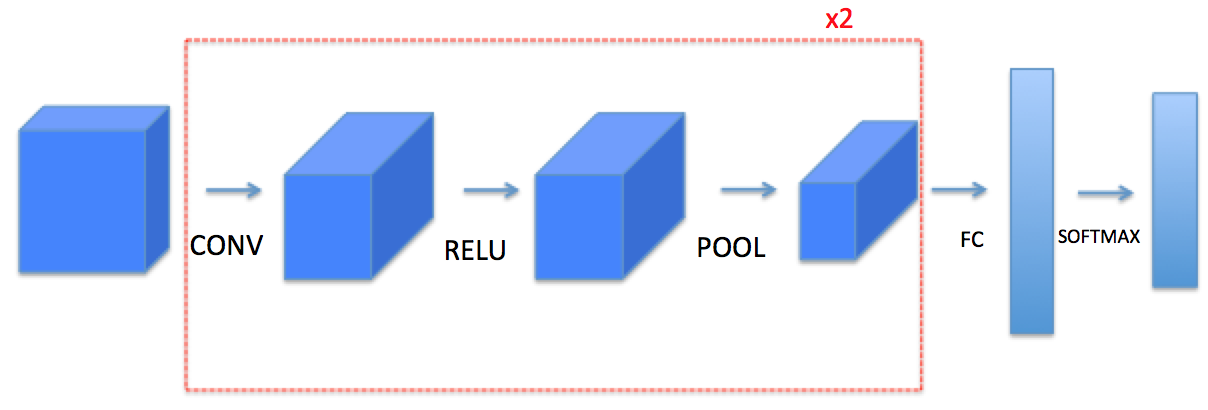
Note: 每一步前向传播,都有对应的 反向传播,因此,你需要把每一步前向传播的parameters,存储到 cache中,用于反向传播.
3. Convolutional Neural Networks
一个卷积层(convolutional layer)将一个输入量转换成不同大小的输出量,如图:
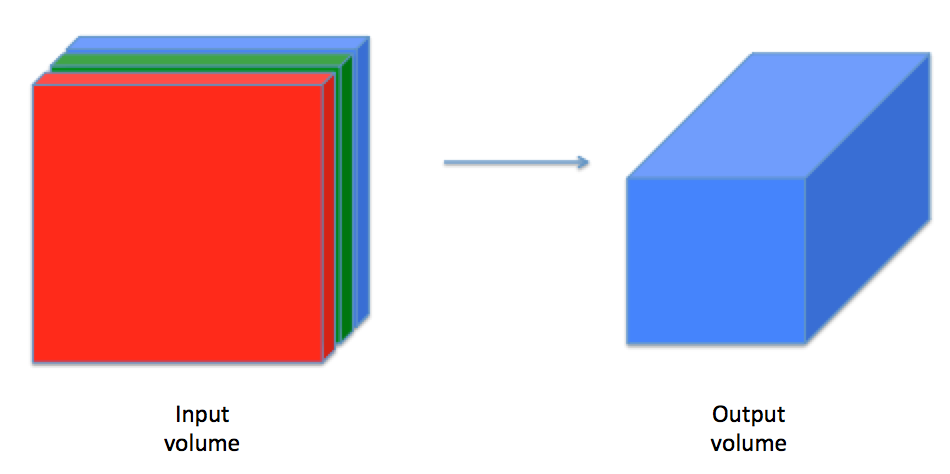
3.1 Zero-Padding
Zero-padding adds zeros around the border of an image:
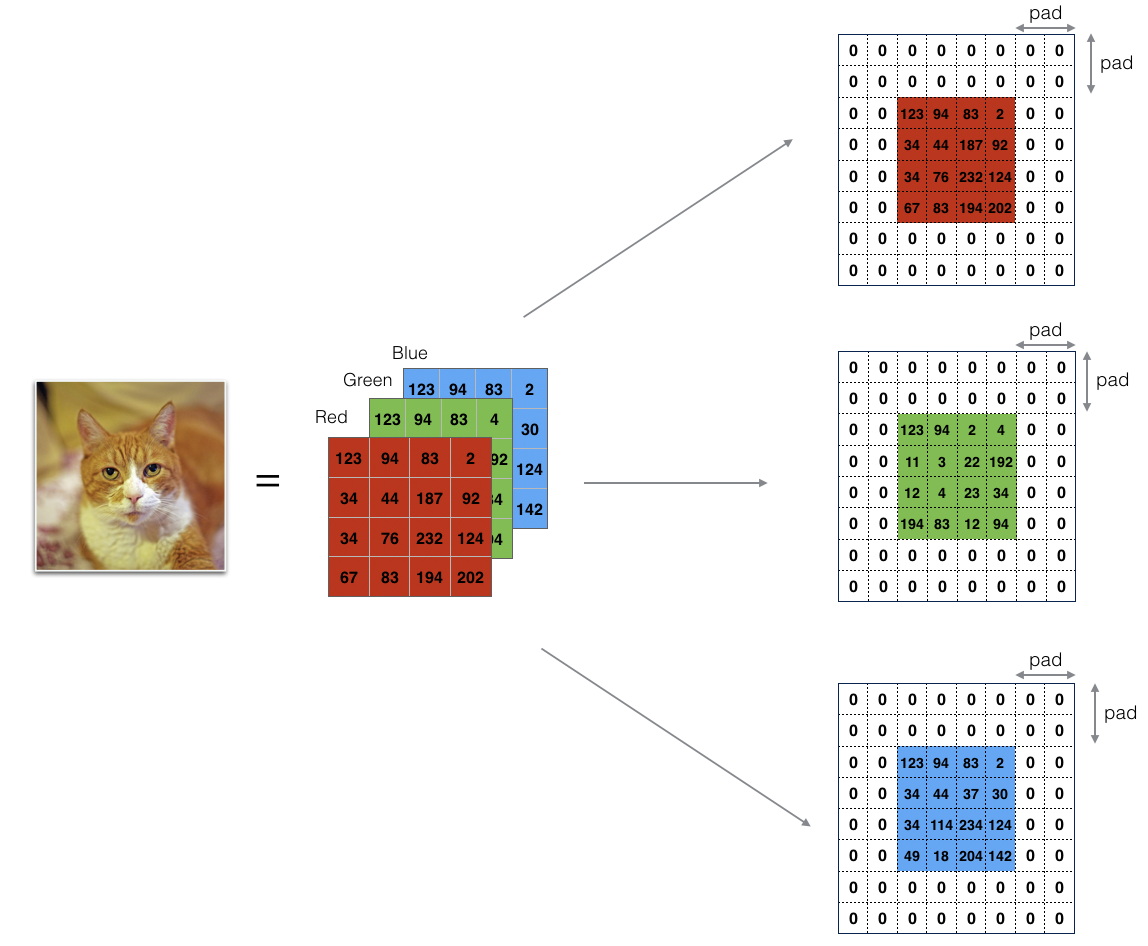
Figure 1 : Zero-Padding:Image (3 channels, RGB) with a padding of 2.
Zero-Padding的两个好处:
-
允许你使用 CONV layer 而不必要减小 the height and width of the volumes.(尤其是搭建深层网络时)(Same convolutions)
-
帮助我们保持图片边缘重要的信息. 没有Padding,很少有值,在下一层能够作为图片的边缘被像素值影响
Exercise:实现函数,用0
填充一批示例X
的所有图像. Note if you want to pad the array "a" of shape ((5,5,5,5,5)) with pad = 1
for the 2nd dimension, pad = 3
for the 4th dimension and pad = 0
for the rest, you would do:
a = np.pad(a, ((0,0), (1,1), (0,0), (3,3), (0,0)), 'constant', constant_values = (..,..))
实现:
# GRADED FUNCTION: zero_pad
def zero_pad(X, pad):
"""
Pad with zeros all images of the dataset X. The padding is applied to the height and width of an image,
as illustrated in Figure 1.
Argument:
X -- python numpy array of shape (m, n_H, n_W, n_C) representing a batch of m images
pad -- integer, amount of padding around each image on vertical and horizontal dimensions
Returns:
X_pad -- padded image of shape (m, n_H + 2*pad, n_W + 2*pad, n_C)
"""
### START CODE HERE ### (≈ 1 line)
# X_pad: (m, n_H + 2*pad, n_W + 2*pad, n_C)
X_pad = np.pad(X, ((0, 0), (pad, pad), (pad, pad), (0, 0)), 'constant', constant_values=0)
### END CODE HERE ###
return X_pad
测试:
np.random.seed(1)
x = np.random.randn(4, 3, 3, 2)
x_pad = zero_pad(x, 2)
print ("x.shape =", x.shape)
print ("x_pad.shape =", x_pad.shape)
print ("x[1,1] =", x[1,1])
print ("x_pad[1,1] =", x_pad[1,1])
fig, axarr = plt.subplots(1, 2)
axarr[0].set_title('x')
axarr[0].imshow(x[0,:,:,0])
axarr[1].set_title('x_pad')
axarr[1].imshow(x_pad[0,:,:,0])
输出:
x.shape = (4, 3, 3, 2)
x_pad.shape = (4, 7, 7, 2)
x[1,1] = [[ 0.90085595 -0.68372786]
[-0.12289023 -0.93576943]
[-0.26788808 0.53035547]]
x_pad[1,1] = [[0. 0.]
[0. 0.]
[0. 0.]
[0. 0.]
[0. 0.]
[0. 0.]
[0. 0.]]
3.2 Single step of convolution
在这一部分中,实现一个卷积的步骤,在该步骤中,将过滤器应用到输入的单个位置中。这将构建卷积单元:
-
需要一个输入volume
-
将滤波器应用到输入的每个位置
-
输出一个不同大小的volume
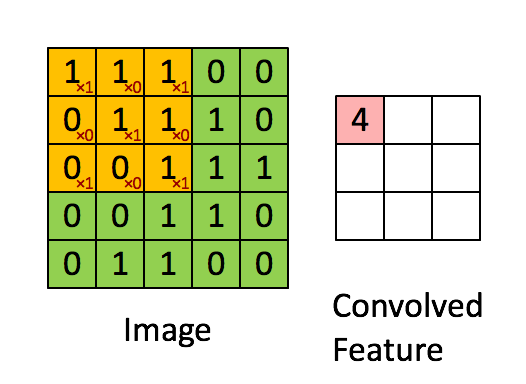
Figure 2 : Convolution operation 2x2的滤波器(filter) 和 步长(stride)为1 (stride = amount you move the window each time you slide)
计算机图像应用中,左边矩阵中的每个值对应于单个像素值,我们通过3x3滤波器与图像卷积,将其值元素与原始矩阵相乘,然后将它们求和并添加偏差。将实现一个卷积步骤,对应于将滤波器应用于其中一个位置以获得单个实值输出。
稍后将将此函数应用于输入的多个位置,以实现完全卷积操作。
Exercise:实现 conv_single_step()
.
# GRADED FUNCTION: conv_single_step
def conv_single_step(a_slice_prev, W, b):
"""
Apply one filter defined by parameters W on a single slice (a_slice_prev) of the output activation
of the previous layer.
Arguments:
a_slice_prev -- slice of input data of shape (f, f, n_C_prev)
W -- Weight parameters contained in a window - matrix of shape (f, f, n_C_prev)
b -- Bias parameters contained in a window - matrix of shape (1, 1, 1)
Returns:
Z -- a scalar value, result of convolving the sliding window (W, b) on a slice x of the input data
"""
### START CODE HERE ### (≈ 2 lines of code)
# Element-wise product between a_slice and W. Do not add the bias yet.
s = np.multiply(a_slice_prev, W)
# Sum over all entries of the volume s.
Z = np.sum(s)
# Add bias b to Z. Cast b to a float() so that Z results in a scalar value.
Z = Z + float(b)
### END CODE HERE ###
return Z
测试:
np.random.seed(1)
a_slice_prev = np.random.randn(4, 4, 3)
W = np.random.randn(4, 4, 3)
b = np.random.randn(1, 1, 1)
Z = conv_single_step(a_slice_prev, W, b)
print("Z =", Z)
输出:
Z = -6.999089450680221
3.3 Convolutional Neural Networks - Forward pass
在前向传播中,你需要很多filters,并在输入上卷积,每次卷积,给你一个2D的矩阵输出,你将stack这些输出,组成一个3D volume:
Exercise: 函数实现 在 input activation A_prev 上卷积filter W.
-
A_prev作为输(上一层
m inputs
激活的输出). 由W表示F filters/weights,b表示bias vector -
其中,每个filter都有自己的bias. 你可以访问包含 stride 和 padding的超参数字典
Hint:
-
在matrix "a_prev"(shape(5,5,3))的左上角,选择一个2x2的slice,你需要:
a_slice_prev = a_prev[0:2,0:2,:]
- 你将使用
start/end
indexes 定义a_slice_prev
- 你将使用
-
要定义 a_slice,你需要首先定义他的corners:
vert_start
,vert_end
,horiz_start
andhoriz_end
,下图展示每个Corner如何用 h,w,f,s 定义:
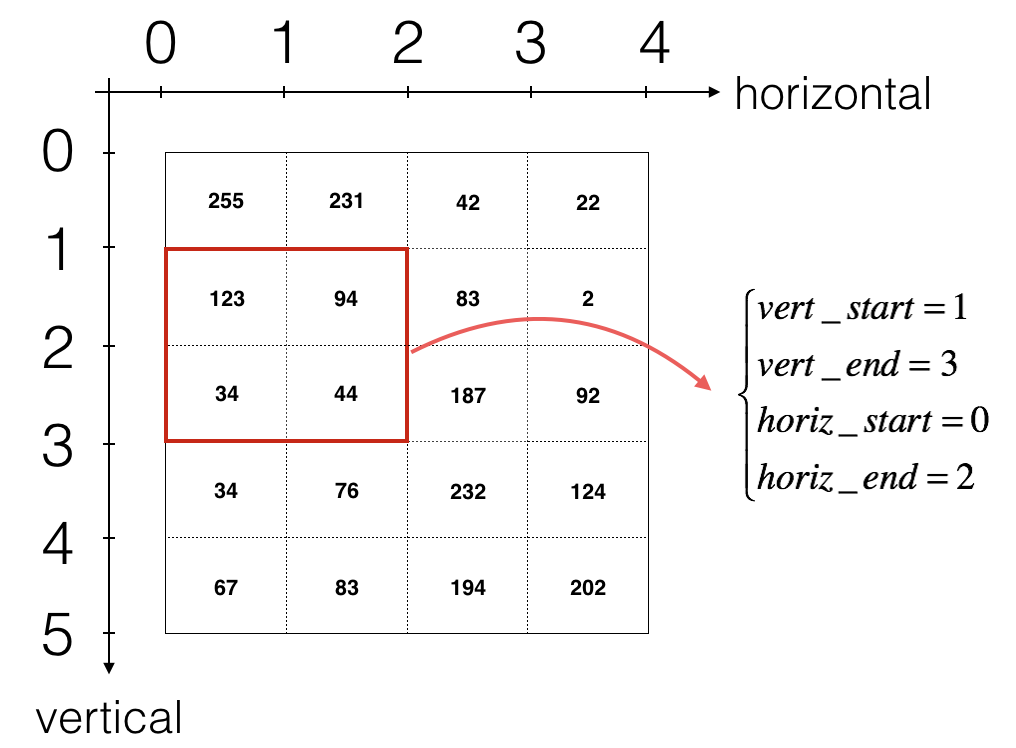
Figure 3 : Definition of a slice using vertical and horizontal start/end (with a 2x2 filter) (This figure shows only a single channel)
Reminder:
卷积后的shape与input shape 有关的公式:
使用for-loop实现:
# GRADED FUNCTION: conv_forward
def conv_forward(A_prev, W, b, hparameters):
"""
Implements the forward propagation for a convolution function
Arguments:
A_prev -- output activations of the previous layer, numpy array of shape (m, n_H_prev, n_W_prev, n_C_prev)
W -- Weights, numpy array of shape (f, f, n_C_prev, n_C)
b -- Biases, numpy array of shape (1, 1, 1, n_C)
hparameters -- python dictionary containing "stride" and "pad"
Returns:
Z -- conv output, numpy array of shape (m, n_H, n_W, n_C)
cache -- cache of values needed for the conv_backward() function
"""
### START CODE HERE ###
# Retrieve dimensions from A_prev's shape (≈1 line)
(m, n_H_prev, n_W_prev, n_C_prev) = A_prev.shape
# Retrieve dimensions from W's shape (≈1 line)
(f, f, n_C_prev, n_C) = W.shape # n_C: n_C个filter
# Retrieve information from "hparameters" (≈2 lines)
stride = hparameters['stride']
pad = hparameters['pad']
# Compute the dimensions of the CONV output volume using the formula given above. Hint: use int() to floor. (≈2 lines)
n_H = int((n_H_prev - f + 2 * pad) / stride) + 1
n_W = int((n_W_prev - f + 2 * pad) / stride) + 1
# Initialize the output volume Z with zeros. (≈1 line)
Z = np.zeros((m, n_H, n_W, n_C)) # n_C: n_C个filter
# Create A_prev_pad by padding A_prev
A_prev_pad = zero_pad(A_prev, pad)
for i in range(m): # loop over the batch of training examples
a_prev_pad = A_prev_pad[i] # Select ith training example's padded activation
for h in range(n_H): # loop over vertical axis of the output volume
for w in range(n_W): # loop over horizontal axis of the output volume
for c in range(n_C): # loop over channels (= #filters) of the output volume
# Find the corners of the current "slice" (≈4 lines)
vert_start = h * stride
vert_end = vert_start + f
horiz_start = w * stride
horiz_end = horiz_start + f
# Use the corners to define the (3D) slice of a_prev_pad (See Hint above the cell). (≈1 line)
a_slice_prev = a_prev_pad[vert_start:vert_end, horiz_start:horiz_end, :]
# Convolve the (3D) slice with the correct filter W and bias b, to get back one output neuron. (≈1 line)
Z[i, h, w, c] = conv_single_step(a_slice_prev, W[...,c], b[...,c]) # 第c个filter的全部W,b
### END CODE HERE ###
# Making sure your output shape is correct
assert(Z.shape == (m, n_H, n_W, n_C))
# Save information in "cache" for the backprop
cache = (A_prev, W, b, hparameters)
return Z, cache
输出:
np.random.seed(1)
A_prev = np.random.randn(10,4,4,3)
W = np.random.randn(2,2,3,8)
b = np.random.randn(1,1,1,8)
hparameters = {"pad" : 2,
"stride": 2}
Z, cache_conv = conv_forward(A_prev, W, b, hparameters)
print("Z's mean =", np.mean(Z))
print("Z[3,2,1] =", Z[3,2,1])
print("cache_conv[0][1][2][3] =", cache_conv[0][1][2][3])
Z's mean = 0.048995203528855794
Z[3,2,1] = [-0.61490741 -6.7439236 -2.55153897 1.75698377 3.56208902 0.53036437
5.18531798 8.75898442]
cache_conv[0][1][2][3] = [-0.20075807 0.18656139 0.41005165]
Finally, CONV layer should also contain an activation, in which case we would add the following line of code:
# Convolve the window to get back one output neuron
Z[i, h, w, c] = ...
# Apply activation
A[i, h, w, c] = activation(Z[i, h, w, c])
You don't need to do it here.
4. Pooling layer
池化层(Pooling layer)减小了输入的height和width,有助于减少计算,并且有助于特征检测在输入位置的不变,两种Pooling Layers:
-
Max-pooling layer: slides an ((f, f)) window over the input and stores the max value of the window in the output.
-
Average-pooling layer: slides an ((f, f)) window over the input and stores the average value of the window in the output.
![]() |
![]() |
池化层(Pooling layers)没有反向传播训练参数,他们有 超参数:window size (f). 它指定计算fxf窗口max or average的 height和width.
4.1 - Forward Pooling
implement MAX-POOL and AVG-POOL, in the same function.
Exercise: Implement the forward pass of the pooling layer. Follow the hints in the comments below.
Reminder:
As there's no padding, the formulas binding the output shape of the pooling to the input shape is:
# GRADED FUNCTION: pool_forward
def pool_forward(A_prev, hparameters, mode = "max"):
"""
Implements the forward pass of the pooling layer
Arguments:
A_prev -- Input data, numpy array of shape (m, n_H_prev, n_W_prev, n_C_prev)
hparameters -- python dictionary containing "f" and "stride"
mode -- the pooling mode you would like to use, defined as a string ("max" or "average")
Returns:
A -- output of the pool layer, a numpy array of shape (m, n_H, n_W, n_C)
cache -- cache used in the backward pass of the pooling layer, contains the input and hparameters
"""
# Retrieve dimensions from the input shape
(m, n_H_prev, n_W_prev, n_C_prev) = A_prev.shape
# Retrieve hyperparameters from "hparameters"
f = hparameters['f']
stride = hparameters['stride']
# Define the dimensions of the output
n_H = int((n_H_prev - f) / stride + 1)
n_W = int((n_W_prev - f) / stride + 1)
n_C = n_C_prev
# Initialize output matrix A
A = np.zeros((m, n_H, n_W, n_C))
### START CODE HERE ###
for i in range(m): # loop over the training examples
for h in range(n_H): # loop on the vertical axis of the output volume
for w in range(n_W): # loop on the horizontal axis of the output volume
for c in range (n_C): # loop over the channels of the output volume
# Find the corners of the current "slice" (≈4 lines)
vert_start = h * stride
vert_end = vert_start + f
horiz_start = w * stride
horiz_end = horiz_start + f
# Use the corners to define the current slice on the ith training example of A_prev, channel c. (≈1 line)
a_prev_slice = A_prev[i, vert_start:vert_end, horiz_start:horiz_end, c]
# Compute the pooling operation on the slice. Use an if statment to differentiate the modes. Use np.max/np.mean.
if mode == "max":
A[i, h, w, c] = np.max(a_prev_slice)
elif mode == "average":
A[i, h, w, c] = np.mean(a_prev_slice)
### END CODE HERE ###
# Store the input and hparameters in "cache" for pool_backward()
cache = (A_prev, hparameters)
# Making sure your output shape is correct
assert(A.shape == (m, n_H, n_W, n_C))
return A, cache
测试:
np.random.seed(1)
A_prev = np.random.randn(2, 4, 4, 3)
hparameters = {"stride" : 2, "f": 3}
A, cache = pool_forward(A_prev, hparameters)
print("mode = max")
print("A =", A)
print()
A, cache = pool_forward(A_prev, hparameters, mode = "average")
print("mode = average")
print("A =", A)
输出:
mode = max
A = [[[[1.74481176 0.86540763 1.13376944]]]
[[[1.13162939 1.51981682 2.18557541]]]]
mode = average
A = [[[[ 0.02105773 -0.20328806 -0.40389855]]]
[[[-0.22154621 0.51716526 0.48155844]]]]
5. Backpropagation in convolutional neural networks
5.1 Convolutional layer backward pass
5.11 Computing dA
- (W_c)是过滤器,(dZ_{hw})是卷积层第h行第w列的使用点乘计算后的输出Z的梯度。
da_prev_pad[vert_start:vert_end, horiz_start:horiz_end, :] += W[:,:,:,c] * dZ[i, h, w, c]
5.1.2 Computing dW
This is the formula for computing (dW_c) ((dW_c) is the derivative of one filter) with respect to the loss:
(dW_c)是一个过滤器的梯度,aslice是(Z_{ij})的激活值
dW[:,:,:,c] += a_slice * dZ[i, h, w, c]
5.1.3 - Computing db:
This is the formula for computing (db) with respect to the cost for a certain filter (W_c):
db[:,:,:,c] += dZ[i, h, w, c]
Exercise: Implement the conv_backward
function below. You should sum over all the training examples, filters, heights, and widths. You should then compute the derivatives using formulas 1, 2 and 3 above.
def conv_backward(dZ, cache):
"""
Implement the backward propagation for a convolution function
Arguments:
dZ -- gradient of the cost with respect to the output of the conv layer (Z), numpy array of shape (m, n_H, n_W, n_C)
cache -- cache of values needed for the conv_backward(), output of conv_forward()
Returns:
dA_prev -- gradient of the cost with respect to the input of the conv layer (A_prev),
numpy array of shape (m, n_H_prev, n_W_prev, n_C_prev)
dW -- gradient of the cost with respect to the weights of the conv layer (W)
numpy array of shape (f, f, n_C_prev, n_C)
db -- gradient of the cost with respect to the biases of the conv layer (b)
numpy array of shape (1, 1, 1, n_C)
"""
### START CODE HERE ###
# Retrieve information from "cache"
(A_prev, W, b, hparameters) = cache
# Retrieve dimensions from A_prev's shape
(m, n_H_prev, n_W_prev, n_C_prev) = A_prev.shape
# Retrieve dimensions from W's shape
(f, f, n_C_prev, n_C) = W.shape
# Retrieve information from "hparameters"
stride = hparameters["stride"]
pad = hparameters["pad"]
# Retrieve dimensions from dZ's shape
(m, n_H, n_W, n_C) = dZ.shape
# Initialize dA_prev, dW, db with the correct shapes
dA_prev = np.zeros((m, n_H_prev, n_W_prev, n_C_prev))
dW = np.zeros((f, f, n_C_prev, n_C))
db = np.zeros((1, 1, 1, n_C))
# Pad A_prev and dA_prev
A_prev_pad = zero_pad(A_prev, pad)
dA_prev_pad = zero_pad(dA_prev, pad)
for i in range(m): # loop over the training examples
# select ith training example from A_prev_pad and dA_prev_pad
a_prev_pad = A_prev_pad[i]
da_prev_pad = dA_prev_pad[i]
for h in range(n_H): # loop over vertical axis of the output volume
for w in range(n_W): # loop over horizontal axis of the output volume
for c in range(n_C): # loop over the channels of the output volume
# Find the corners of the current "slice"
vert_start = h * stride
vert_end = vert_start + f
horiz_start = w * stride
horiz_end = horiz_start + f
# Use the corners to define the slice from a_prev_pad
a_slice = a_prev_pad[vert_start:vert_end, horiz_start:horiz_end, :]
# a_slice = A_prev_pad[i, vert_start:vert_end, horiz_start:horiz_end, :]
# Update gradients for the window and the filter's parameters using the code formulas given above
da_prev_pad[vert_start:vert_end, horiz_start:horiz_end, :] += W[:,:,:,c] * dZ[i, h, w, c]
# dA_prev_pad[i, vert_start:vert_end, horiz_start:horiz_end, :] += W[:,:,:,c] * dZ[i, h, w, c]
dW[:,:,:,c] += a_slice * dZ[i, h, w, c]
db[:,:,:,c] += dZ[i, h, w, c]
# Set the ith training example's dA_prev to the unpaded da_prev_pad (Hint: use X[pad:-pad, pad:-pad, :])
dA_prev[i, :, :, :] = da_prev_pad[pad:-pad, pad:-pad, :]
# dA_prev[i, :, :, :] = dA_prev_pad[i, pad:-pad, pad:-pad, :]
### END CODE HERE ###
# Making sure your output shape is correct
assert(dA_prev.shape == (m, n_H_prev, n_W_prev, n_C_prev))
return dA_prev, dW, db
测试:
np.random.seed(1)
dA, dW, db = conv_backward(Z, cache_conv)
print("dA_mean =", np.mean(dA))
print("dW_mean =", np.mean(dW))
print("db_mean =", np.mean(db))
dA_mean = 1.4524377775388075
dW_mean = 1.7269914583139097
db_mean = 7.839232564616838
5.2 Pooling layer - backward pass
5.2.1 Max pooling - backward pass
创建掩码矩阵(保存最大值位置)
ps: 4是最大值,则mask中相应位置为1; 其他值不是最大值,则为0
Exercise: Implement create_mask_from_window()
. This function will be helpful for pooling backward.
Hints:
- np.max() may be helpful. It computes the maximum of an array.
- If you have a matrix X and a scalar x:
A = (X == x)
will return a matrix A of the same size as X such that:
A[i,j] = True if X[i,j] = x
A[i,j] = False if X[i,j] != x
- Here, you don't need to consider cases where there are several maxima in a matrix.
def create_mask_from_window(x):
"""
Creates a mask from an input matrix x, to identify the max entry of x.
Arguments:
x -- Array of shape (f, f)
Returns:
mask -- Array of the same shape as window, contains a True at the position corresponding to the max entry of x.
"""
### START CODE HERE ### (≈1 line)
mask = (x == np.max(x))
### END CODE HERE ###
return mask
测试:
np.random.seed(1)
x = np.random.randn(2,3)
mask = create_mask_from_window(x)
print('x = ', x)
print("mask = ", mask)
x = [[ 1.62434536 -0.61175641 -0.52817175]
[-1.07296862 0.86540763 -2.3015387 ]]
mask = [[ True False False]
[False False False]]
Why do we keep track of the position of the max?
-
It's because this is the input value that ultimately influenced the output, and therefore the cost.
-
Backprop is computing gradients with respect to the cost, so anything that influences the ultimate cost should have a non-zero gradient.
-
So, backprop will "propagate" the gradient back to this particular input value that had influenced the cost.
5.2.2 Average pooling - backward pass
均值池化层的反向传播:
This implies that each position in the (dZ) matrix contributes equally to output because in the forward pass, we took an average.
Exercise: Implement the function below to equally distribute a value dz through a matrix of dimension shape. Hint
def distribute_value(dz, shape):
"""
Distributes the input value in the matrix of dimension shape
Arguments:
dz -- input scalar
shape -- the shape (n_H, n_W) of the output matrix for which we want to distribute the value of dz
Returns:
a -- Array of size (n_H, n_W) for which we distributed the value of dz
"""
### START CODE HERE ###
# Retrieve dimensions from shape (≈1 line)
(n_H, n_W) = shape
# Compute the value to distribute on the matrix (≈1 line)
average = dz / (n_H * n_W)
# Create a matrix where every entry is the "average" value (≈1 line)
a = np.ones(shape) * average
### END CODE HERE ###
return a
测试:
a = distribute_value(2, (2,2))
print('distributed value =', a)
distributed value = [[0.5 0.5]
[0.5 0.5]]
5.2.3 Putting it together: Pooling backward
compute backward propagation on a pooling layer.
Exercise: Implement the pool_backward
function in both modes ("max"
and "average"
).
-
You will once again use 4 for-loops (iterating over training examples, height, width, and channels).
-
You should use an
if/elif
statement to see if the mode is equal to'max'
or'average'
. -
If it is equal to 'average' you should use the
distribute_value()
function you implemented above to create a matrix of the same shape asa_slice
. -
Otherwise, the mode is equal to '
max
', and you will create a mask withcreate_mask_from_window()
and multiply it by the corresponding value of dZ.
def pool_backward(dA, cache, mode = "max"):
"""
Implements the backward pass of the pooling layer
Arguments:
dA -- gradient of cost with respect to the output of the pooling layer, same shape as A
cache -- cache output from the forward pass of the pooling layer, contains the layer's input and hparameters
mode -- the pooling mode you would like to use, defined as a string ("max" or "average")
Returns:
dA_prev -- gradient of cost with respect to the input of the pooling layer, same shape as A_prev
"""
### START CODE HERE ###
# Retrieve information from cache (≈1 line)
(A_prev, hparameters) = cache
# Retrieve hyperparameters from "hparameters" (≈2 lines)
stride = hparameters['stride']
f = hparameters['f']
# Retrieve dimensions from A_prev's shape and dA's shape (≈2 lines)
m, n_H_prev, n_W_prev, n_C_prev = A_prev.shape
m, n_H, n_W, n_C = dA.shape
# Initialize dA_prev with zeros (≈1 line)
dA_prev = np.zeros(A_prev.shape)
for i in range(m): # loop over the training examples
# select training example from A_prev (≈1 line)
a_prev = A_prev[i]
for h in range(n_H): # loop on the vertical axis
for w in range(n_W): # loop on the horizontal axis
for c in range(n_C): # loop over the channels (depth)
# Find the corners of the current "slice" (≈4 lines)
vert_start = h * stride
vert_end = vert_start + f
horiz_start = w * stride
horiz_end = horiz_start + f
# Compute the backward propagation in both modes.
if mode == "max":
# Use the corners and "c" to define the current slice from a_prev (≈1 line)
a_prev_slice = a_prev[vert_start:vert_end, horiz_start:horiz_end, c]
# a_prev_slice = A_prev[i, vert_start:vert_end, horiz_start:horiz_end, c]
# Create the mask from a_prev_slice (≈1 line)
mask = create_mask_from_window(a_prev_slice)
# Set dA_prev to be dA_prev + (the mask multiplied by the correct entry of dA) (≈1 line)
dA_prev[i, vert_start:vert_end, horiz_start:horiz_end, c] += np.multiply(mask, dA[i, h, w, c])
elif mode == "average":
# Get the value a from dA (≈1 line)
da = dA[i, h, w, c]
# Define the shape of the filter as fxf (≈1 line)
shape = (f, f)
# Distribute it to get the correct slice of dA_prev. i.e. Add the distributed value of da. (≈1 line)
dA_prev[i, vert_start:vert_end, horiz_start:horiz_end, c] += distribute_value(da, shape)
### END CODE ###
# Making sure your output shape is correct
assert(dA_prev.shape == A_prev.shape)
return dA_prev
测试:
np.random.seed(1)
A_prev = np.random.randn(5, 5, 3, 2)
hparameters = {"stride" : 1, "f": 2}
A, cache = pool_forward(A_prev, hparameters)
dA = np.random.randn(5, 4, 2, 2)
dA_prev = pool_backward(dA, cache, mode = "max")
print("mode = max")
print('mean of dA = ', np.mean(dA))
print('dA_prev[1,1] = ', dA_prev[1,1])
print()
dA_prev = pool_backward(dA, cache, mode = "average")
print("mode = average")
print('mean of dA = ', np.mean(dA))
print('dA_prev[1,1] = ', dA_prev[1,1])
mode = max
mean of dA = 0.14571390272918056
dA_prev[1,1] = [[ 0. 0. ]
[ 5.05844394 -1.68282702]
[ 0. 0. ]]
mode = average
mean of dA = 0.14571390272918056
dA_prev[1,1] = [[ 0.08485462 0.2787552 ]
[ 1.26461098 -0.25749373]
[ 1.17975636 -0.53624893]]